mmWave-based Activity Recognition
Overview
Motivation & Objective
Human Activity Recognition (HAR) has a wide range of real-world applications, such as health care and fitness tracking. Device-based approaches for HAR (e.g. smart watches) have limitations due to cost and discomfort. Many significant efforts have recently been made to explore device-free HAR that utlizes the information collected from wireless infrastructures (e.g. WiFi signals). Other existing wireless devices, such as cameras, can potentially leak and lead to privacy issues. We propose a a network, utlizing mmWave data that can accurately classify amongst different human activities, that is cheaper and user-friendly.
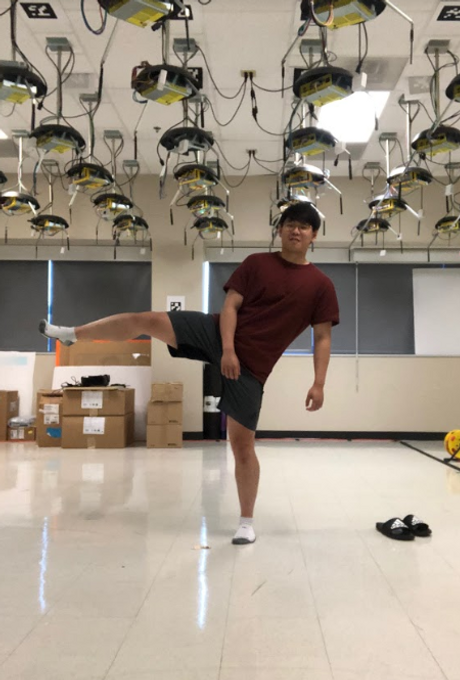
Why mmWaves?
mmWaves are a class of radar technology that uses short wavelength electromagnetic waves. Radar systems transmit these waves and capture reflected signals from objects in their path. By using these reflected signals, we can then determine the range, velocity, and angle of objects. We decided to use mmWaves for this project because of its numerous advantages. One of the advantages of using mmWaves is the size of its components. The components to process mmWaves waves, such as the antennas, are relatively small which is beneficial. Another advantage of using mmWaves is that they provide a high accuracy because they transmit high frequency signals. mmWaves have the ability to detect movements that are as small as a fraction of a millimeter. In addition, every set of mmWave data that we collected is unique for each activity. This is especially beneficial because it can allow us to classify between different activities accurately. We can use these signals along with FFT and machine learning algorithms for classification.
